Interpolation#
Assume, we have a function \(y(x)\) that is sampled at \(n\) discrete points \({x_1, x_2, \cdots, x_n}\) with high accuracy
This data could be obtained from experiments or simulations (e.g., FDM, FEM)
How to evaluate \(y(x^*)\) where \(x_i < x^* < x_j\) (\(1\le i \lt j\le n\))?
Use interpolation
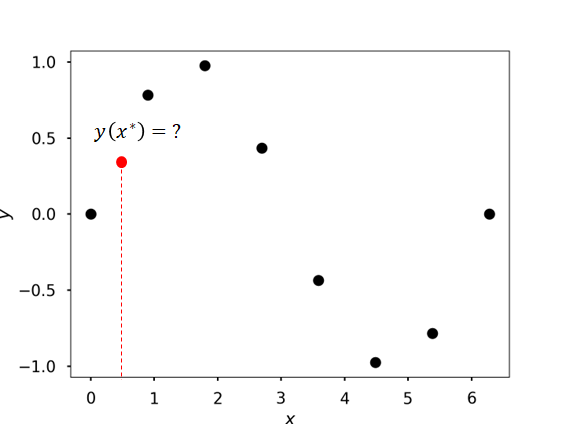
Interpolation is the process of contructing a function \(\hat{y}(x)\) that is exact at the sampled points and approximates the function elsewhere
\(\hat{y}(x)\) is the interpolation function
Interpolation Types#
Piecewise constant
Linear
Quadratic
Cubic spline
Higher-order splines
…
Piecewise constant interpolation of data sampled from \(sin(x)\)
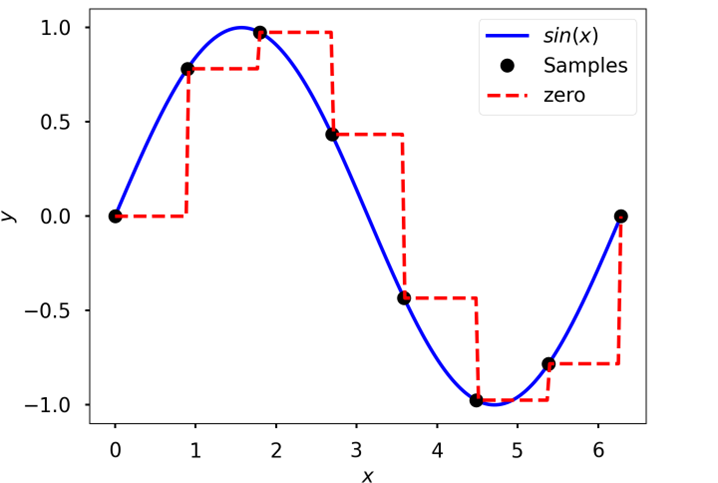
Linear interpolation of data sampled from \(sin(x)\)
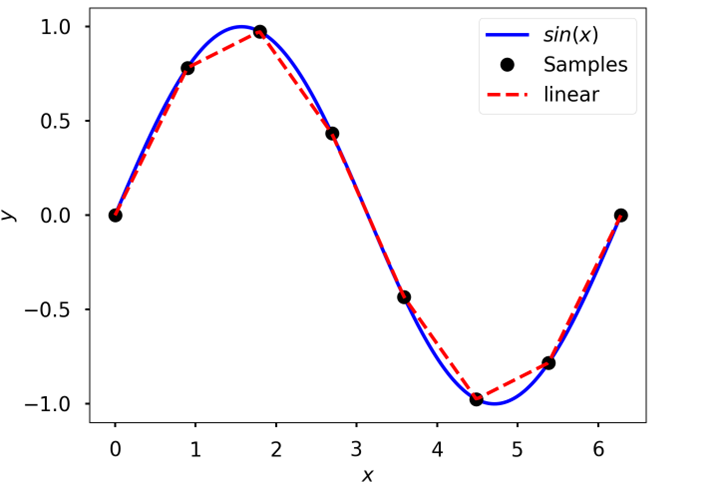
Cubic interpolation of data sampled from \(sin(x)\)
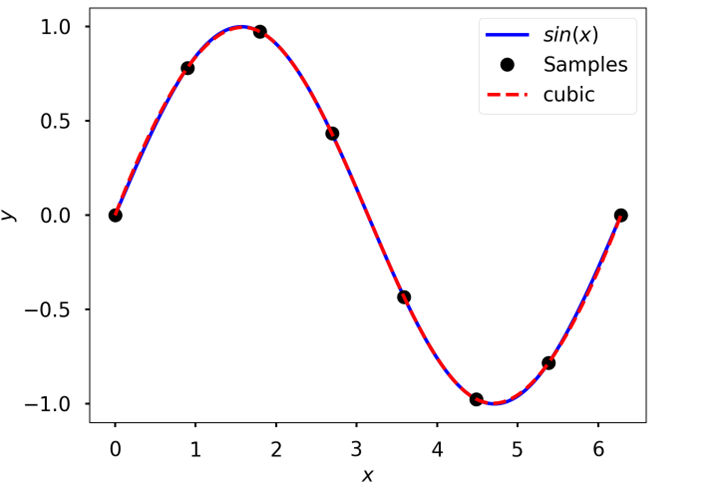
Key assumption: input data has adequate information to construct an interpolation function that is sufficiently accurate
Fewer samples \(\implies\) poor interpolation
Interpolation with four samples (left) and three samples (right)
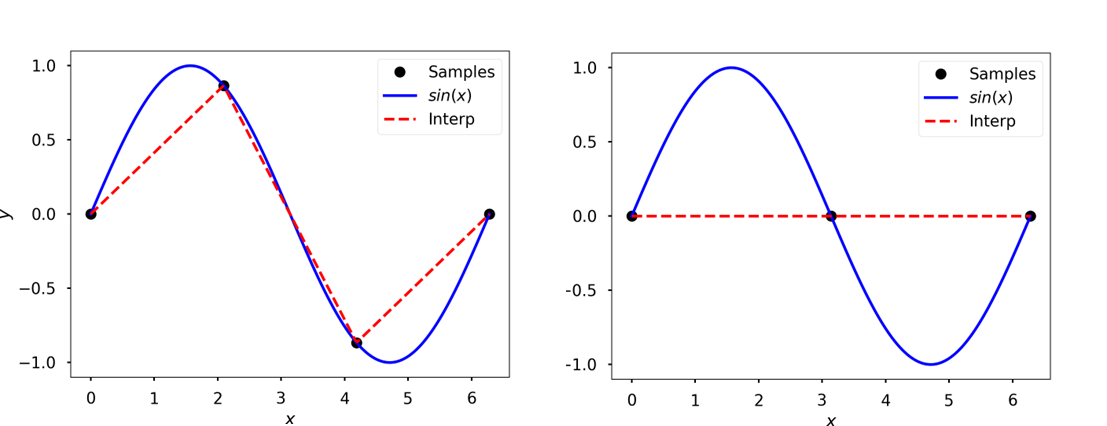
The scipy sub-module
interpolate
provides classes for interpolationUnivariate interpolation (1D data)
interp1d
InterpolatedUnivariateSpline
\(\cdots\)
Multivariate interpolation
2D data
interp2d
BivariateSpline
and derived classes\(\cdots\)
\(n\)D data
interpn
Refer to documentation for more details
Example#
Find an interpolation function for sampled data from \(sin(x)\)
%matplotlib widget
import numpy as np
from scipy import interpolate
import matplotlib.pyplot as plt
# Sampled data from sin
xs = np.linspace(0, 2*np.pi, 8)
ys = np.sin(xs)
---------------------------------------------------------------------------
ModuleNotFoundError Traceback (most recent call last)
Cell In[1], line 1
----> 1 get_ipython().run_line_magic('matplotlib', 'widget')
2 import numpy as np
3 from scipy import interpolate
File /opt/hostedtoolcache/Python/3.11.9/x64/lib/python3.11/site-packages/IPython/core/interactiveshell.py:2480, in InteractiveShell.run_line_magic(self, magic_name, line, _stack_depth)
2478 kwargs['local_ns'] = self.get_local_scope(stack_depth)
2479 with self.builtin_trap:
-> 2480 result = fn(*args, **kwargs)
2482 # The code below prevents the output from being displayed
2483 # when using magics with decorator @output_can_be_silenced
2484 # when the last Python token in the expression is a ';'.
2485 if getattr(fn, magic.MAGIC_OUTPUT_CAN_BE_SILENCED, False):
File /opt/hostedtoolcache/Python/3.11.9/x64/lib/python3.11/site-packages/IPython/core/magics/pylab.py:103, in PylabMagics.matplotlib(self, line)
98 print(
99 "Available matplotlib backends: %s"
100 % _list_matplotlib_backends_and_gui_loops()
101 )
102 else:
--> 103 gui, backend = self.shell.enable_matplotlib(args.gui.lower() if isinstance(args.gui, str) else args.gui)
104 self._show_matplotlib_backend(args.gui, backend)
File /opt/hostedtoolcache/Python/3.11.9/x64/lib/python3.11/site-packages/IPython/core/interactiveshell.py:3665, in InteractiveShell.enable_matplotlib(self, gui)
3662 import matplotlib_inline.backend_inline
3664 from IPython.core import pylabtools as pt
-> 3665 gui, backend = pt.find_gui_and_backend(gui, self.pylab_gui_select)
3667 if gui != None:
3668 # If we have our first gui selection, store it
3669 if self.pylab_gui_select is None:
File /opt/hostedtoolcache/Python/3.11.9/x64/lib/python3.11/site-packages/IPython/core/pylabtools.py:338, in find_gui_and_backend(gui, gui_select)
321 def find_gui_and_backend(gui=None, gui_select=None):
322 """Given a gui string return the gui and mpl backend.
323
324 Parameters
(...)
335 'WXAgg','Qt4Agg','module://matplotlib_inline.backend_inline','agg').
336 """
--> 338 import matplotlib
340 if _matplotlib_manages_backends():
341 backend_registry = matplotlib.backends.registry.backend_registry
ModuleNotFoundError: No module named 'matplotlib'
# Cubic spline interpolation
f1 = interpolate.interp1d(xs, ys, kind = 'cubic')
# Alternate approach
f2 = interpolate.InterpolatedUnivariateSpline(xs, ys, k = 3)
f1
andf2
are both callable objects and can be treated as any other Python function
Using the interpolation function beyond the bounds of the sampled data will cause an error
# Interpolated data
x = np.linspace(0, 2*np.pi, 100)
y1 = f1(x)
y2 = f2(x)
# Plot
fig, axs = plt.subplots(2, 1)
axs[0].plot(x, y1, '-r', label='interp1d')
axs[1].plot(x, y2, '-r', label='UnivariateSpline')
for ax in axs:
ax.plot(xs, ys, 'ok', markersize=6, label='Samples')
ax.plot(x, np.sin(x), '--b', label='sin(x)')
ax.legend();
Curve Fitting#
Given noisy data, what is the best mathematical model (i.e., function) that fits the data?
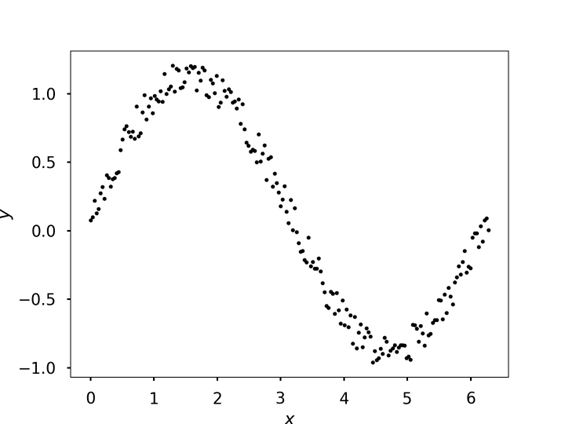
Least squares fitting, or regression, is a common approach
Minimizes the squared error in the predicted values of the best fit \(\hat{y}\) and the actual values \(y\)
The unknown model can be written as:
Here, \(f\) is a known function with \(a_0, a_1, \cdots, a_j\) being unknown coefficients to be determined by minimizing the error \(E\)
If the mathematical model \(f\) is a polynomial, it is referred to as linear regression
Curve fitting in numpy and scipy
np.linalg.lstsq
Works for mathematical models of the form: \( \hat{y}(x) = \sum\limits_{i=0}^n a_i f_i(x)\)
Minimizing the error in this case is equivalent to solving a linear system with \(a_i\) being the unknowns
Fitting with polynomial functions
np.polynomial
subpackage provides modules for working special polynomial functions likePolynomial
Chebyshev
Lagrange
Legendre
Laguerre
\(\cdots\)
Fitting with splines
scipy.interpolate.UnivariateSpline
class
Fitting with
scipy.optimize
optimize.curve_fit
which internally usesoptimize.least_squares
Allows non-linear least squares fit
Example#
Fit data sampled from \(sin\) with added noise
# Seed for random numbers
np.random.seed(2412023)
# Data with noise - typically obtained from experiments
x = np.linspace(0, 2*np.pi, 200)
y = np.sin(x) + 0.25*np.random.rand(len(x))
Curve fitting with the model \(\hat{y}(x) = a_0 + a_1 sin(x) + a_2 cos(x)\)
For an arbitrary point \(x_i\) with a function value of \(y_i\), we have:
This gives us \(n\) equations in \(a_0, a_1\), and \(a_2\) (\(n\) = number of samples)
# Least squares fit
A = np.vstack([np.ones_like(x),
np.sin(x),
np.cos(x)]).T
fit = np.linalg.lstsq(A, y)
fit[0] # ignore the warning
# Fitted curve
y_fit = fit[0][0] + fit[0][1]*np.sin(x) + fit[0][2]*np.cos(x)
# Plot
def plot_fit(x_fit, y_fit, lbl='Fit'):
fig, ax = plt.subplots()
ax.plot(x, y, 'ok', markersize=2, label="Samples")
ax.plot(x_fit, y_fit, '-r', label=lbl)
ax.set(xlabel='x', ylabel='y')
ax.legend();
plot_fit(x, y_fit, "Least Squares Fit")
Fit with a 6th degree polynomial
# Least squares polynomial fit of order 6
fitPoly = np.polynomial.Polynomial.fit(x, y, 6)
print(fitPoly)
# Plot
x_fit, y_fit = fitPoly.linspace()
plot_fit(x_fit, y_fit, "Polynomial Fit")
Fit with a 6th degree Laguerre polynomials
# Least squares Laguerre polynomial fit of order 6
fitLaguerre = np.polynomial.Laguerre.fit(x, y, 6)
print(fitLaguerre)
# Plot
x_fit, y_fit = fitLaguerre.linspace()
plot_fit(x_fit, y_fit, "Laguerre Poly Fit")
Fit with a spline function
# Spline fitting
fitSpline = interpolate.UnivariateSpline(x, y)
# Interpolation function
yHat = fitSpline(x)
# Plot
plot_fit(x, yHat, "Spline fit")
Fitting using the
optimize
sub-moduleNeeds a prescribed mathematical model
Unlike the
linalg.lstsq
function, the model can be non-linear
from scipy import optimize
# Model function - can be non-linear
def model_func(x, a0, a1, k1, a2, k2):
return a0 + a1*np.sin(k1*x) + a2*np.cos(k2*x)
# Fit
optPar, covPar = optimize.curve_fit(model_func, x, y)
optPar # Optimized parameters
# Plot
y_fit = model_func(x, optPar[0], optPar[1], optPar[2], optPar[3], optPar[4])
plot_fit(x, y_fit, "Nonlinear least squares fit")